Discipline the Baby
(proposal)
Multi-interface installation, projection in space, parallel running AI model, action analysis illustration, action sheet, infant action design diagram, infant 3D animation rendering, dimensions variable, 2023
Discipline the Baby is a multi-interface scheme of work that incorporates advanced technologies such as machine learning, AI-generated image models and interfaces for conversations with large language models. A key element is the application of artificial intelligence in analyzing human movements, which involves a complex process of regulation and re-regulation in order to allow the AI to generate human movements to train humans again.
In the process of human-machine interaction, they influence and constrain each other. The complexity of this human-machine interaction is demonstrated in the work. Humans train the AI to analyze body movements, and the AI generates corresponding movements, creating a unique collaborative relationship between the two parties.
Further, the work discusses the human-computer relationship in a future perspective, which is achieved by controlling the movements and behaviors of a newborn baby. A baby is controlled by an AI model that has been trained to control its movements and behaviors, a process that demonstrates the importance of disciplining and re-disciplining, as well as the interplay of power between humans and machines and algorithms that play off each other. This dynamic change of power is reflected in the work, and people can become the disciplined objects.
Through a combination of multi-screen projection interface, machine learning and human-machine interaction, this new media artwork presents an exploratory and experimental visual experience that provokes the viewer to think about the interrelationship between technology and human beings. It aims to explore the modes of interaction between artificial intelligence and humans, and to provoke discussions on social, ethical and power issues arising from technological development.
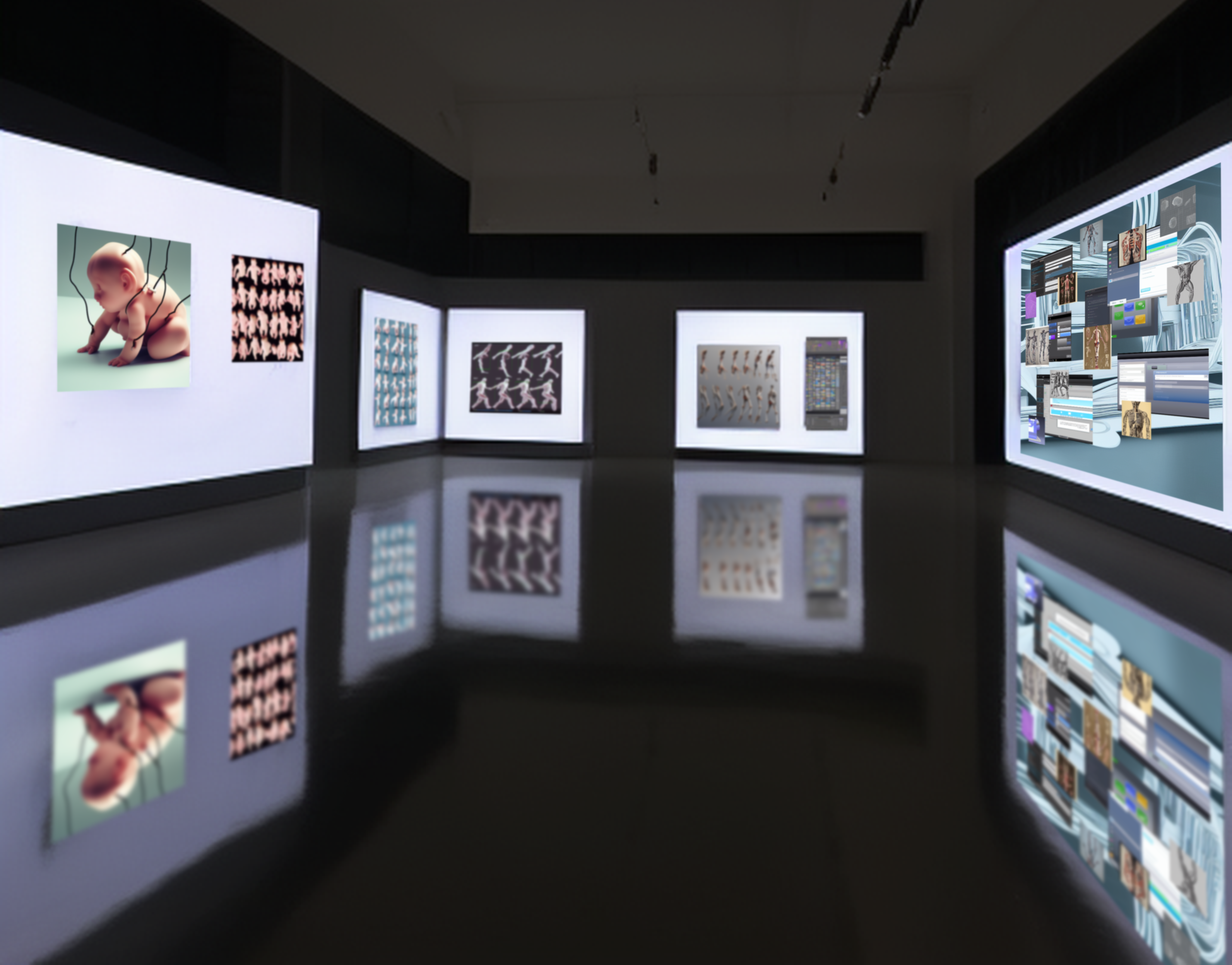
installation view ↑
* The project is in the proposal stage and is open for further development
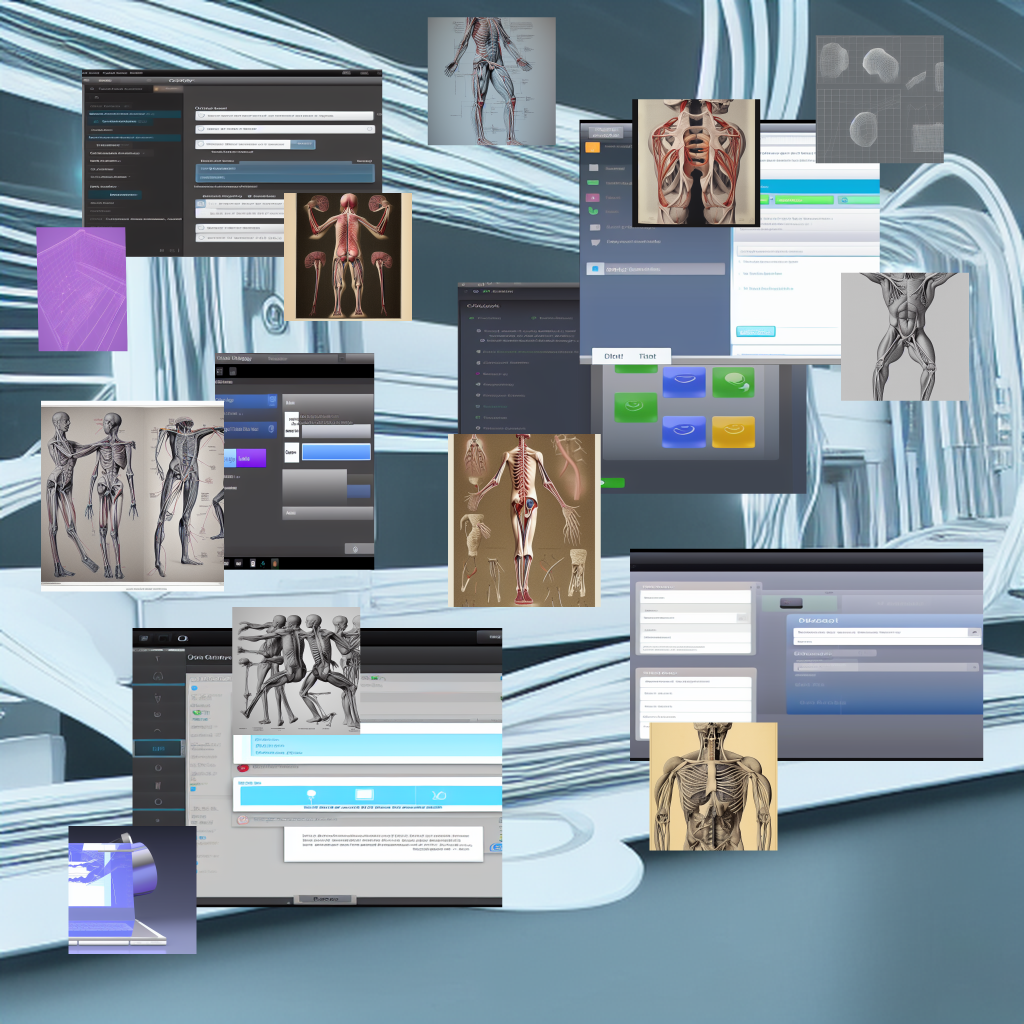
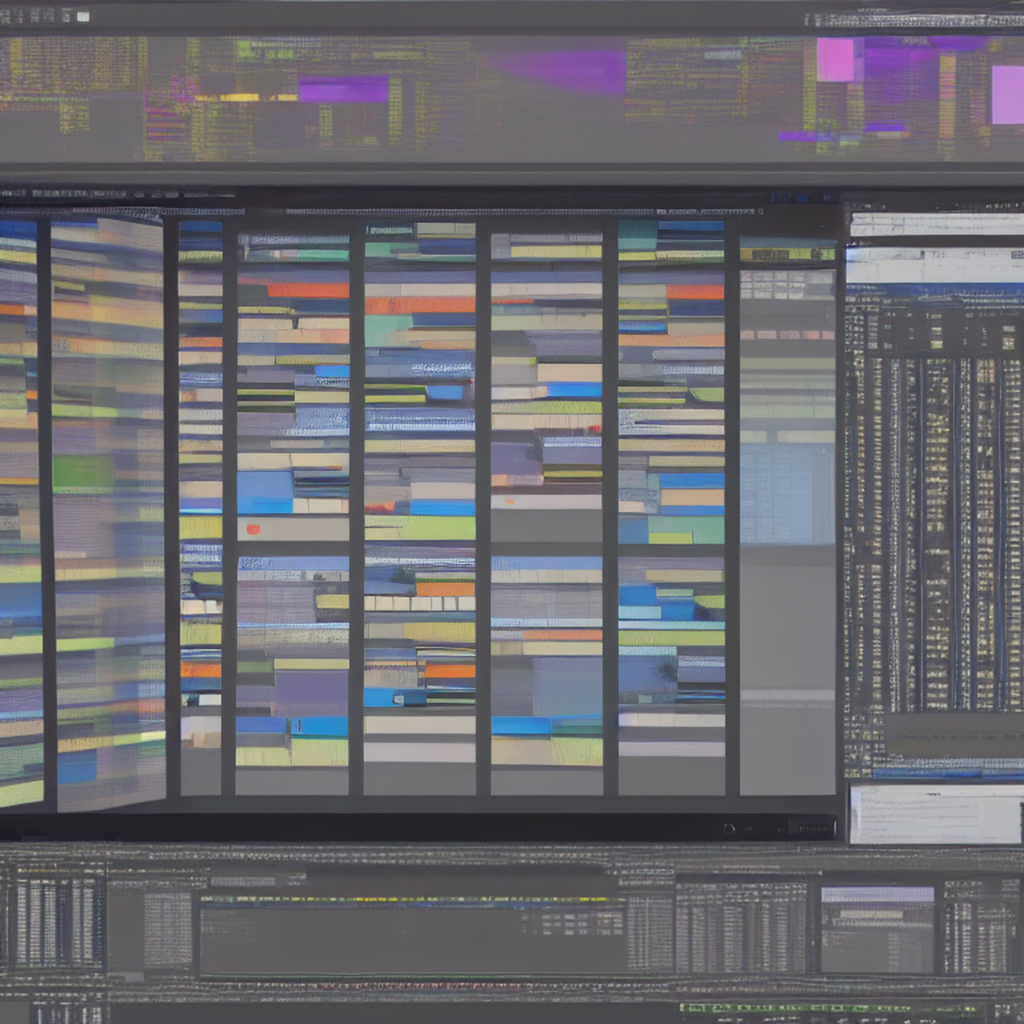
Interface 1/2
Machine Learning: Using machine learning techniques, a model is trained to analyze and understand the constituent elements of human action. This can be achieved through supervised learning using labeled action datasets. The model can learn information about the trajectory of human skeletal joints, postural changes, and associations between joints.
AI-generated image models: The learned action components are transformed into realistic human action images by AI-generated image models. This can be achieved by generating images of the corresponding skeletal structure, muscle morphology, and human posture. The generated model can use deep learning techniques, combined with trained model parameters, to transform the learned action elements into a visual image representation.
Large language model dialogue interface: When users interact with the artwork, a platform for communication can be provided through the large language model dialogue interface. The user can engage in a dialogue with the system to ask questions about the composition of the action or to perform an interpretation of the action elements. The Big Language Model can use natural language processing and generation techniques to understand the user’s questions and give relevant answers or explanations.
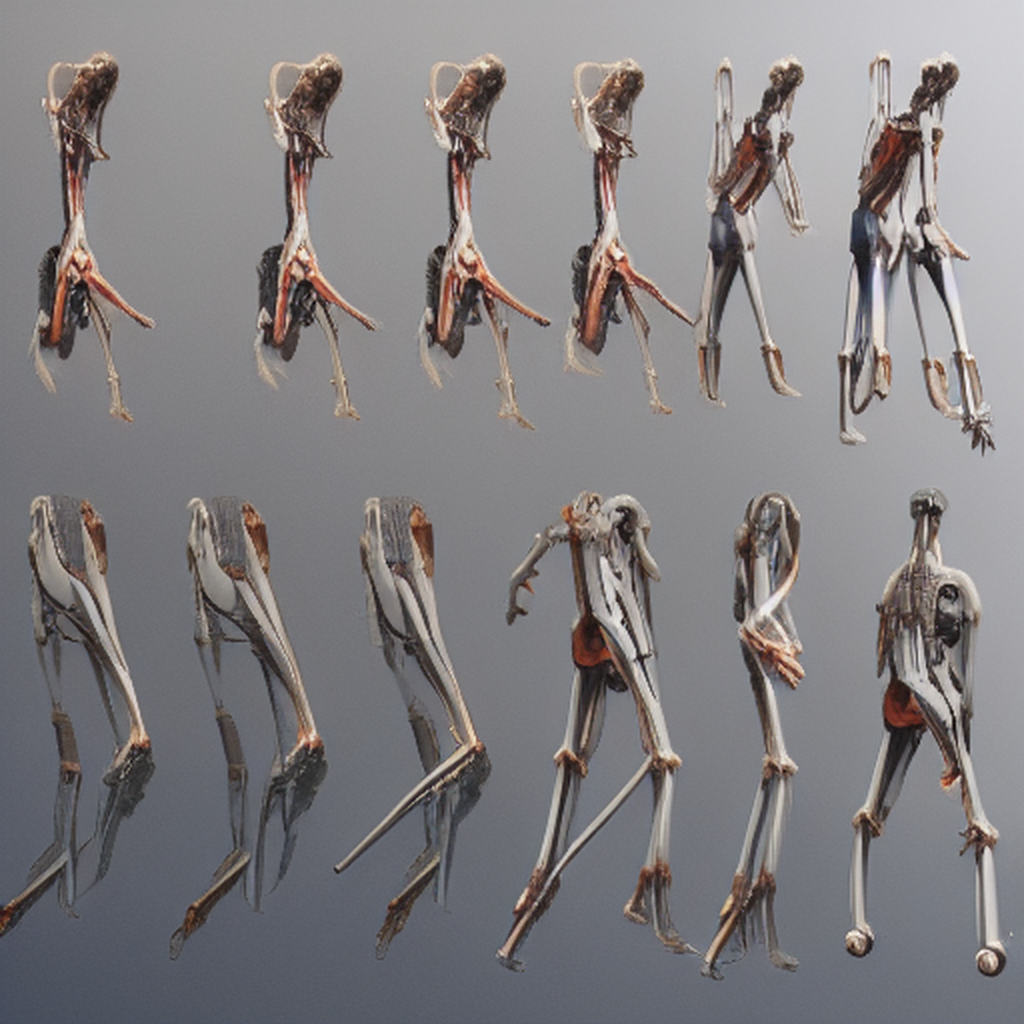
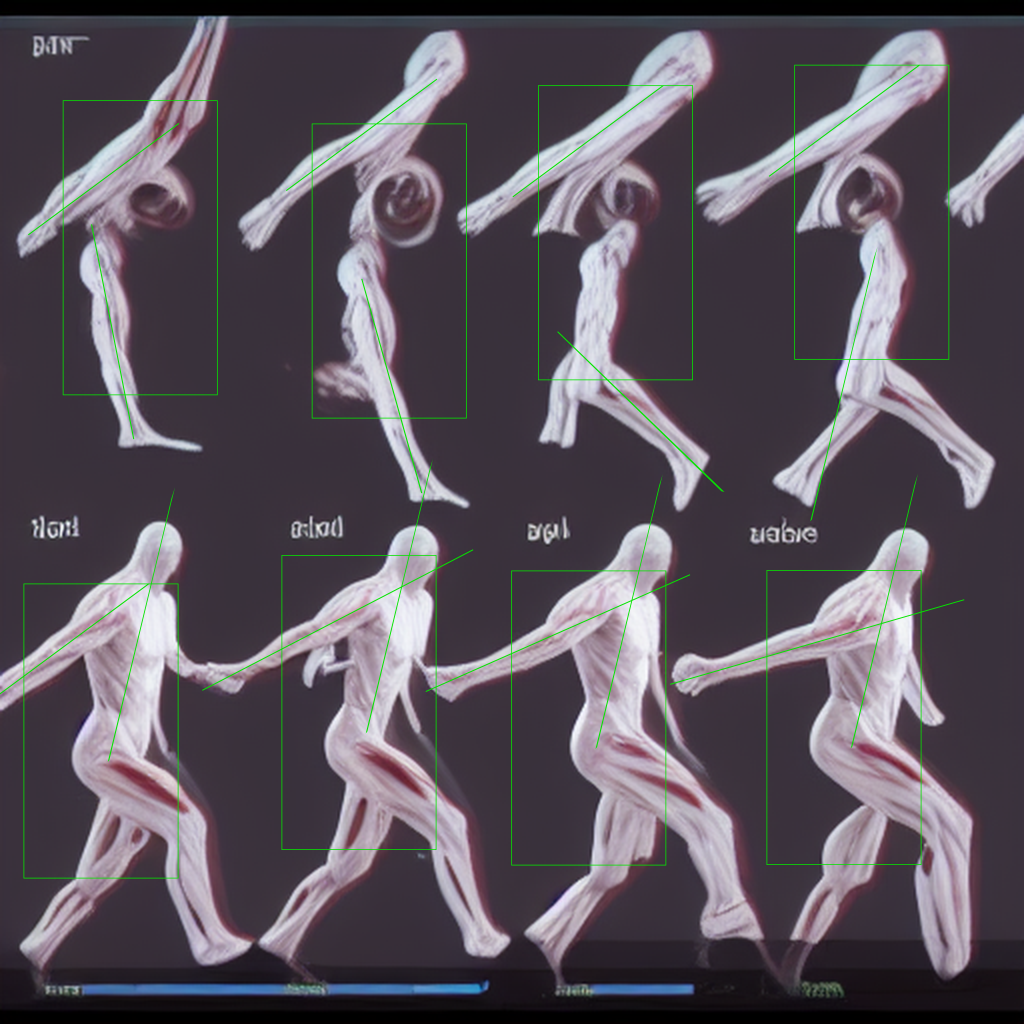
Interface 3/4
The input image or video is analyzed and processed using machine learning algorithms and image recognition techniques. This can include using convolutional neural networks (CNNs) or other deep learning models to extract image features, detect human joints and postures, track movements, and more. By training these models, they can learn how to recognize and understand key elements in human movements.
Based on the understanding and analysis of human actions, image generation techniques are used to generate the corresponding images. This can be achieved using generative models such as Generative Adversarial Networks (GAN) or Variational Autoencoders (VAE). The generative models can learn the input action elements and use the learned patterns to generate realistic images of human actions. These models can generate images that contain details such as skeletal structure, muscle morphology, and human pose.
In order to train machine learning and image generation models, a large annotated dataset needs to be prepared. This can be a human action database containing various actions and poses, where each sample has the corresponding action label and key point location information. Using these datasets, machine learning models can be trained by supervised learning while adversarial training is performed using generative models to generate realistic images.
Through the techniques of machine learning and image recognition, the system is able to understand and analyze the components of human action. The generative model uses these learned elements to generate the corresponding human action images. The whole process requires large amounts of data and computational resources, and combines advanced deep learning algorithms and generative models to achieve high quality results for action analysis and image generation.
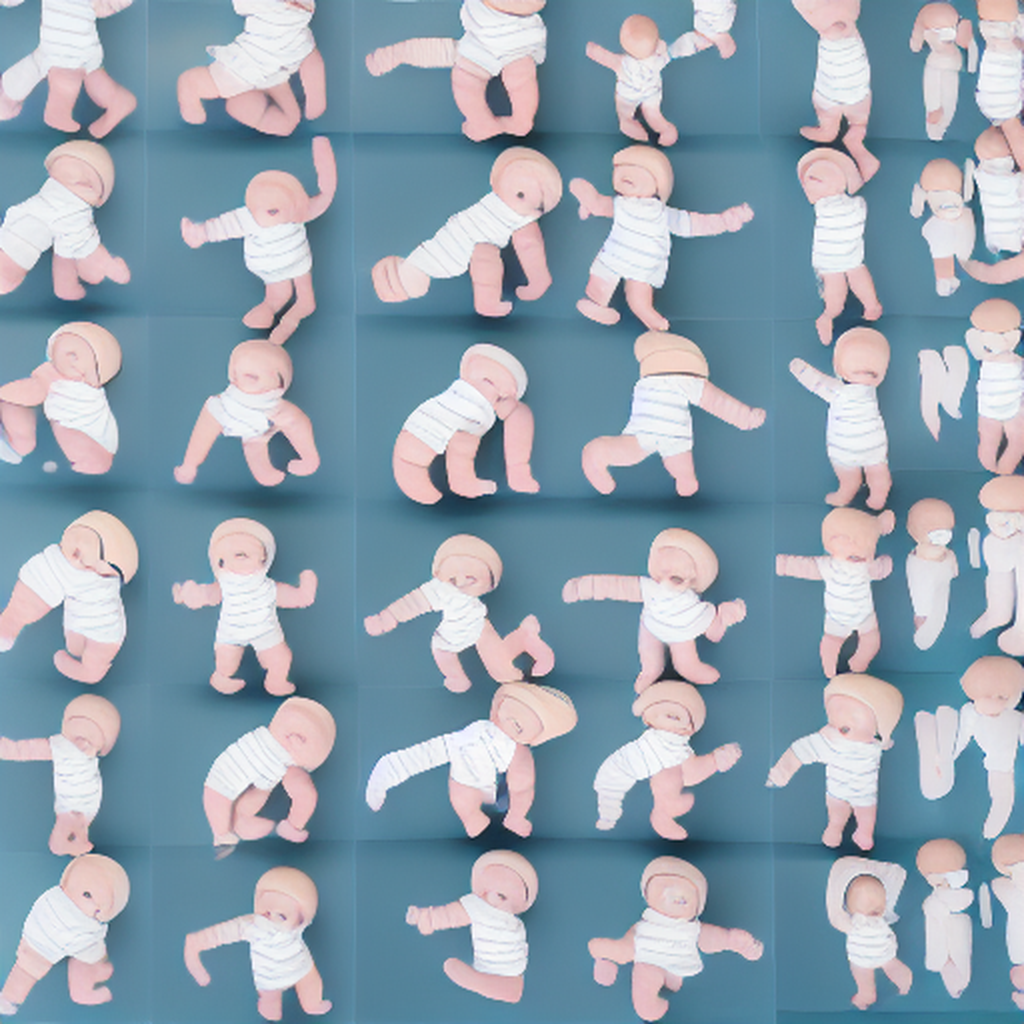
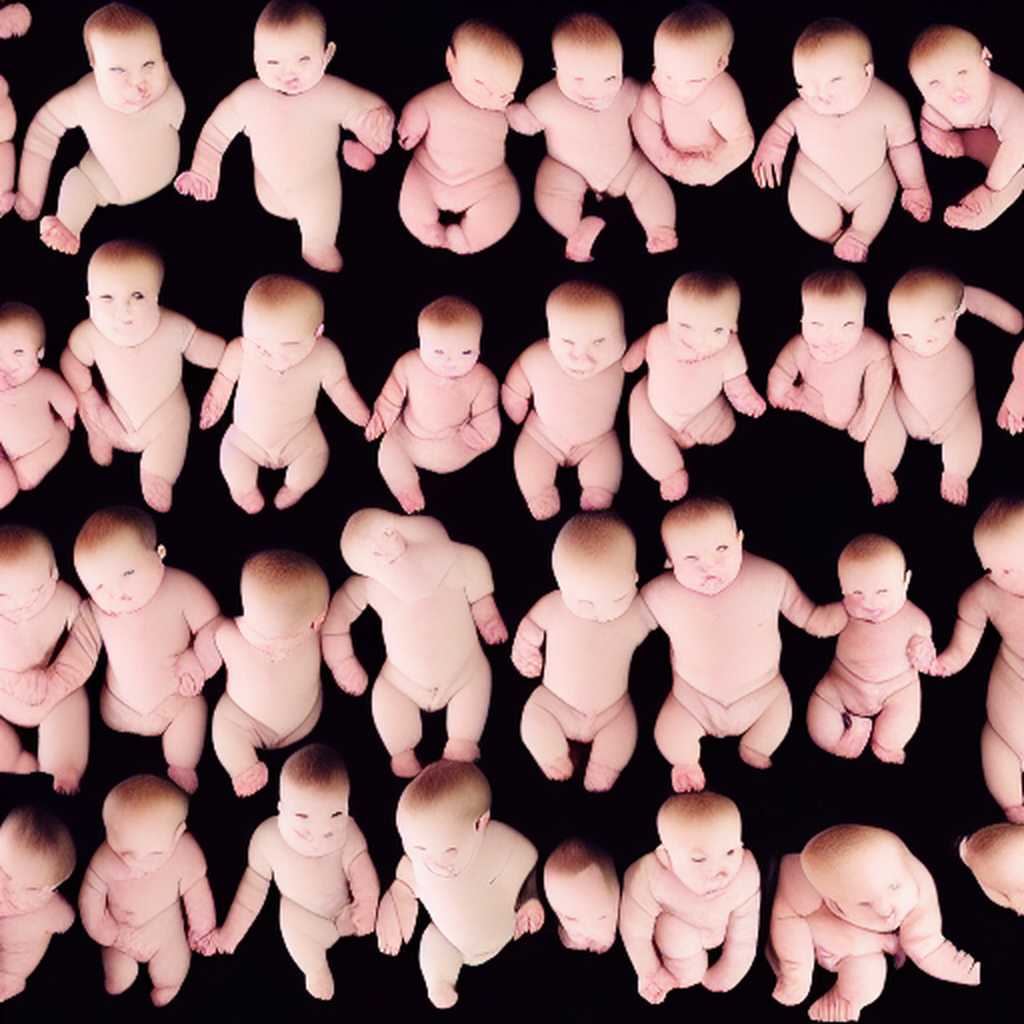
Interface 5/6
Through in-depth analysis and pattern extraction of data from motion analysis, important features and patterns in human behavior patterns can be identified. This can include trajectories of human joint movements, temporal sequences of movements, postural changes, etc. Through these analyses, detailed information about human behavior patterns can be obtained.
Designing action templates: Based on the obtained behavior pattern data, some action templates are designed as a reference for newborns. These action templates can include different action types and time sequences to demonstrate the diversity and complexity of human behavior patterns. When designing movement templates, the physiological characteristics and developmental stages of newborns should be considered to ensure that the movements are beneficial to their physical abilities and development.
In addition, the process of regulation and re-regulation is performed by feeding the movement templates into a machine learning system. The system can adjust the movement templates based on the feedback and adaptation of the newborn to better suit the physical characteristics and developmental needs of the newborn. This involves optimization and iteration of the action generation model and machine learning algorithms to achieve good interaction and adaptability with the newborn.
Human-computer interaction and feedback mechanisms are taken into account in the design of the actions. A two-way interaction can be established between the newborn and the system, and feedback from the newborn can be obtained by means of sensing devices and touch sensors to adjust and improve the generated action templates. This feedback mechanism can help the system to better understand the needs of the newborn and further optimize the action design.
Power relations are intertwined and evolve through the process of AI’s regulation of the newborn and vice versa. the AI, as a technological tool and designer, exerts influence and control over the newborn through its capabilities and rule making. However, the newborn, as a subject with its own characteristics and individual differences, provides feedback and adjustment to the AI through feedback and interaction. This intersection of power relations reflects the complex interaction between man and machine, rules and individuals, and provokes thoughts and discussions on the redistribution of technological power and social ethics.
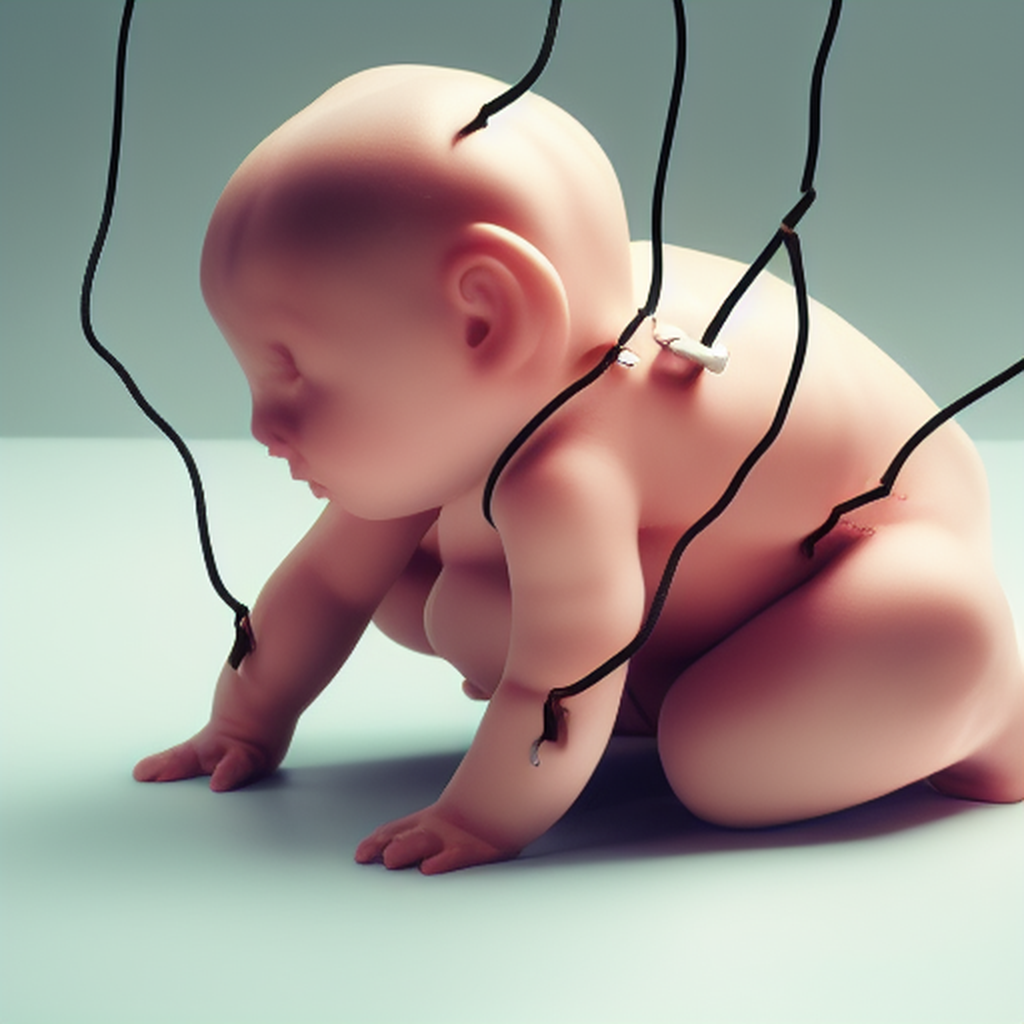
Interface 7
The infant’s movements are simulated using a previously established movement analysis model. The model can infer the movements the infant is attempting by monitoring the infant’s joint movements and postural changes.
Based on the output of the motion analysis model, a motion chart is generated. The movement chart can be a time series of data describing the infant’s movement state and posture at different points in time. The chart can contain information about the infant’s joint angles, body trajectory, etc. for subsequent animation generation.
Based on the generated action charts, CG animations of the infant doing the actions are created using computer graphics and animation creation software. The infant’s body structure and pose can be modeled and rendered in a virtual environment based on the data in the chart. Through frame-by-frame animation techniques, the virtual infant is allowed to perform the corresponding actions in the CG environment based on the time-series data in the action chart.
During the CG animation process, attention can be paid to the details and performance of the movements. According to the data in the action chart, the details of joint movements, muscle deformation and body posture of the virtual baby are optimized to make it more realistic and natural. At the same time, attention is paid to expressing the unique softness of infants and the limits of movement amplitude in order to maintain the realism and credibility of the animation.